Data has become a game changer within the last decade. Knowing how to collect, use, and apply it can quite literally dictate a business’ performance and, more importantly, its relationship with its customers.
If you choose to discover and use key insights from your data, you’re able to understand your customers’ needs better and offer them the appropriate services. Otherwise, you inevitably fall into a “spray and pray” mentality - you send out a generalized marketing campaign and hope that something will stick to someone. As a result, your customer relations don’t necessarily strengthen - if anything, they will weaken over time if you continue to throw irrelevant content at them.
But this all seems little wishy-washy. To put everything into a bit more context, let’s take a look at one of the most successful retailers in the world - Target. Target’s decision to use data to both identify its customer segments and personalize its advertising efforts truly revolutionized the way data could be used in the retail space.
Target’s Pregnancy Prediction Algorithm
In 2008, Target hired statistician Andrew Pole. His primary goal was to maximize sales by identifying patterns in consumer behavior.
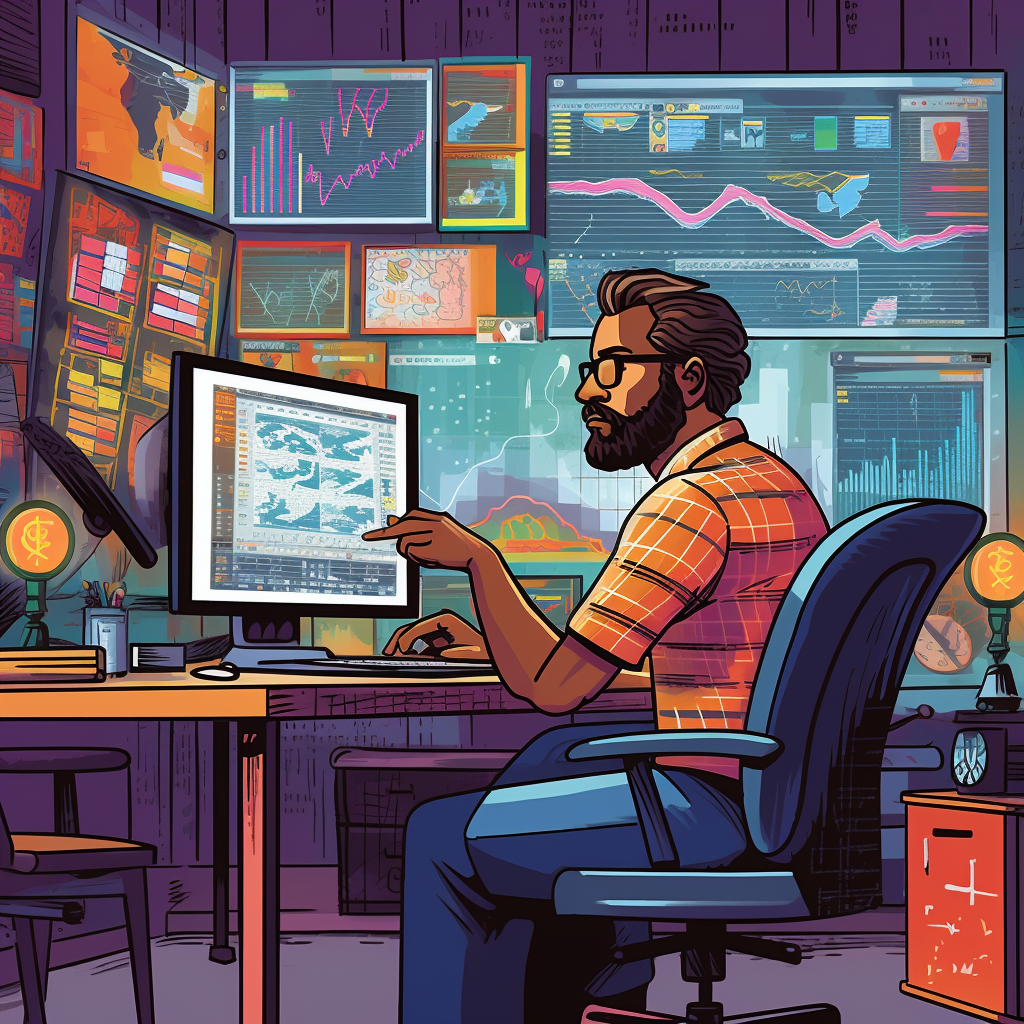
For the record, Target already had a system built to collect data on every single one of its customers. Each customer had a unique ID, assigned to track a plethora of different data points, ranging from age to salary to relationship status. Don’t forget that Target also could buy – and did buy – customer information, too.
So Pole took all of that data and helped Target’s marketing team understand consumer behavior.
Sounds pretty standard, so far. Until it wasn’t anymore.
Pole’s brilliant. Amazing. Never seen before. He’s – I’ll stop, sorry, the data nerd in me was fanboying. But you know who else was excited by his work? Target’s marketing team.
Seeing the improvements and strides Pole was making, they were ecstatic to jump on that train. Target sells literally everything, and their new goal was to make Target the only store consumers needed to go to for anything. But Target doesn’t have infinite supply, so they have to be smart with their inventory. That means understanding consumer behavior wasn’t enough – they had to predict it, too.
As Pole recalls to Charles Duhigg, a former NYT columnist and senior editor, two marketing colleagues came up to him and popped the question that launched Target’s data mining scheme: “If we wanted to figure out if a customer is pregnant based on shopping trends, could we do that?”
Why expecting mothers? 2 reasons:
- There is a clear timeline. Pregnancies roughly last 9 months, with that time being split into trimesters.
- Clear material needs throughout the timeline. As a pregnancy progresses, a mother will need to buy specific things to care for herself and/or the baby.
The thing is, Target needed to figure out who was an expecting mother and what those “clear material needs” were. That’s Pole’s cue.
With Pole’s nifty skills and statistical models, they were able to detect certain behavioral patterns. A few examples include:
- Unscented lotion purchases peaked around the start of the second trimester.
- Hand sanitizers and scent-free soap purchases surged closer to a mother’s delivery date.
- At the start of the pregnancy (within the first 20 weeks), women would buy more vitamins and supplements.
These observations were more than just markers. Given this information and a consumer’s purchasing habits, Pole could determine whether or not a woman was pregnant. If the answer was yes, he could even predict their due date within a small margin of error.
To demonstrate how accurate Pole’s “pregnancy prediction” algorithm was, here’s a quick story on how Target managed to predict a teen daughter’s pregnancy before her parents even knew:
A year after Pole’s algorithm was implemented, a father had discovered a coupon book sent to his daughter that shocked him. In that coupon book were several suggestions for baby strollers and other pregnancy items. The father was in disbelief, speeding to his local target and insisting they had made a mistake.
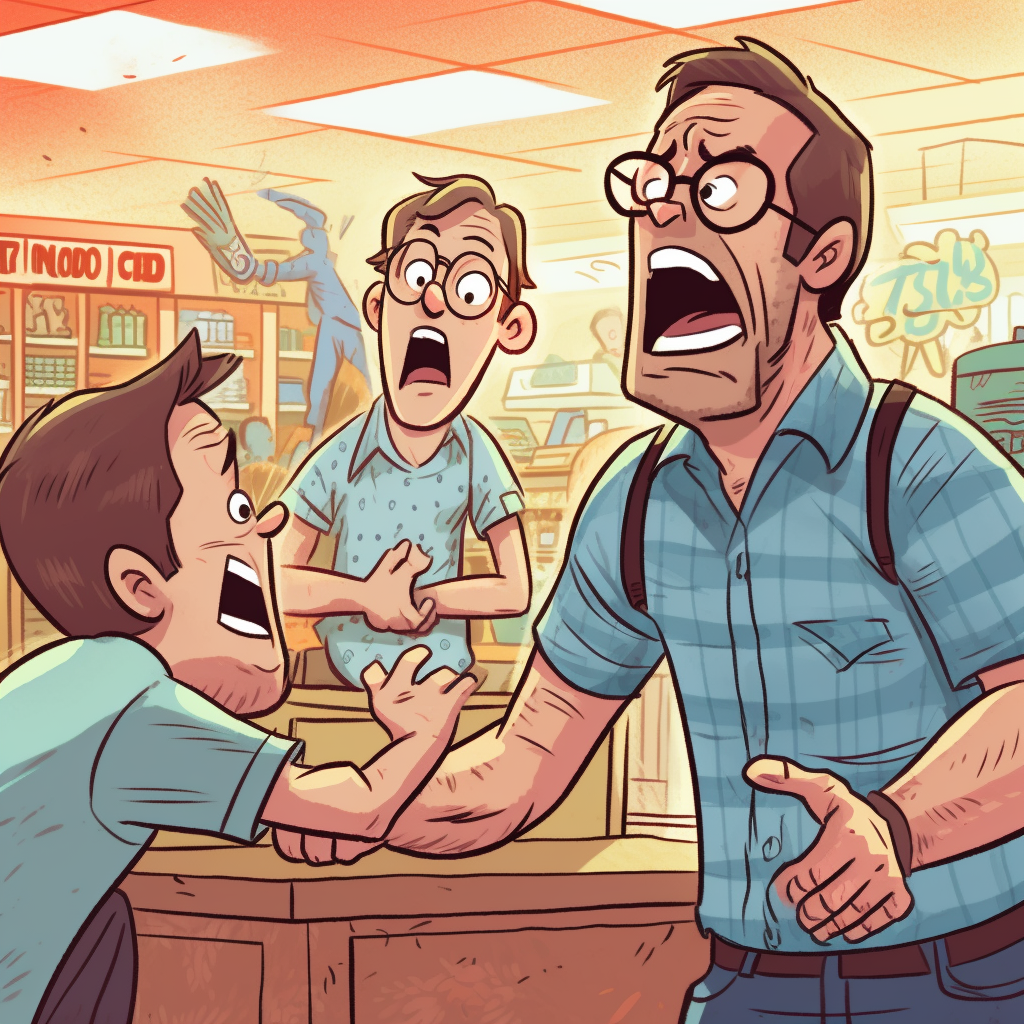
Turns out, a week later, the father phoned that same Target to apologize. His daughter was indeed expecting, and Target had found out before him.
Bridging Together Customer Segmentation and Personalization
For marketers, this case study is a perfect of how, given the right datasets and insights, you can know your customers at this intimate of a level. Not only can you figure out what kinds of customers you have, but you can determine the buying and spending habits of each segment and appeal to each accordingly.
In this case, Pole’s algorithm was applied as a recommendation-based engine. In the good ol’ days, Target mailed coupon booklets to their customers’ homes, and by personalizing them to each individual customer, they could make the most out of both their inventory while maximizing sales.
Whether or not Pole’s work was the main driver in skyrocketing Target’s revenue from $44B to $67B within 8 years is besides the point. The true takeaway is the logic behind Pole’s prediction algorithm.
The algorithm relies heavily on both customer segmentation and predictive analytics, but more than that, it’s the use of the algorithm to take that customer segmentation data and create directed campaigns to appeal to all of Target’s customer base.
If anything, Pole’s pregnancy prediction tool enabled the company to become a trendsetter in personalized ads.
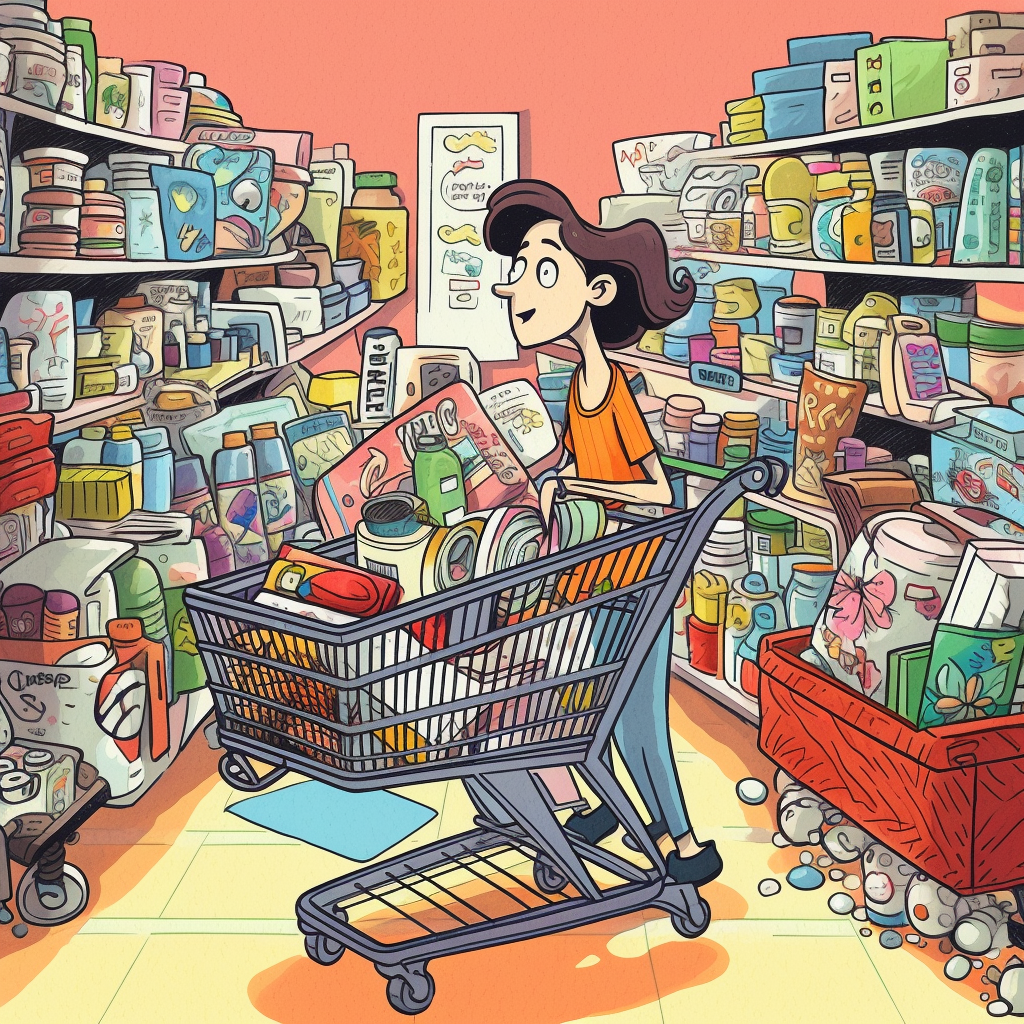
Target was able to persuade its customers to use them for everything. Instead of only buying a few specific necessities from Target, customers were beginning to unintentionally buy just about everything as they began to use their personalized coupons. If a mother had to go in-store to buy a stroller, then she might as well use those discounts they got for zinc supplements and a new bath rug. Oh, and a men’s polo shirt? Might as well pick that up for her husband since there was a random coupon for that – wouldn’t want to let that go to waste!
Similarly, with the help of Cotera, not only can we pull out the most important strands of information from your data, but we can also help you figure out how to use and apply that information in the most effective way. Whether that means identifying your customer segments or personalizing your next marketing strategy or both, the insights you get is crucial to strengthening your relationship with your customers.
Your shtick doesn’t have to be personalized ads. But the point is, customer segmentation is your starting point, while personalization is your end goal. It is crucial for you to understand your customers’ distinct behaviors and develop the right marketing tactics to connect the dots.