I know someone who, every week, DoorDashes a bottle of Martinelli’s sparkling cider. (She has shelves displaying the empty bottles to prove it.) As a business, customers like these make it incredibly easy to guess their next purchase day, which is exactly what it sounds like: the next time that a consumer is expected to buy a product.
But what if the pattern of purchasing for a consumer isn’t so often or regular as my Martinelli’s-obsessed friend’s? Estimating the time that elapses before a customer buys something again can be useful for optimizing marketing strategies, like sending that promotional email at the perfect time—but as always, a good guess requires some data analysis, especially when it comes to the industry of e-commerce. Let’s break down the analysis process so we can elevate your business’s email game!
Methods to Predict the Next Purchase Day
First, let’s consider some general steps without the intricacies of data.
- A natural first step is analyzing the past purchase behavior of the consumer: when have they bought items in the past? Is this a regular enough pattern to forecast future purchases? This can look like a log of past purchase dates, the quantity of what they bought, the price of the purchase, or even the type of device that the purchase was made on.
- You can also look at the specific products of interest: are they, like my friend’s Martinellis, items you can expect to run out within a week? Are they monthly shopping trips? Or are they bi-annual splurges, like vacations? The type of product can help inform your analysis, even if the customer’s behavior is irregular or not fully documented.
- To make even more informed predictions, you can add in some details: do you have a consumer’s birthday on record? You can probably expect their purchases to spike around the holidays, anniversaries, or any other similar special events.
Once you have these pieces of information, you can combine them to refine your approach to marketing. For example, if you know that a consumer is only purchasing your product every few weeks, there’s not much value derived from sending them weekly reminders. If you’re certain that a consumer is loyal to you, perhaps you don’t need to send that offer to entice them to buy. But if there’s no purchase in the time frame that you expect, maybe you should remind them of the great deals you have. (Or refine your data…)
Using Simple Math to Predict Purchases
Now, let’s add in some math! At the most basic level, suppose you have four data points for a single customer:
- Purchase 1: March 3
- Purchase 2: March 17
- Purchase 3: March 29
- Purchase 4: April 17
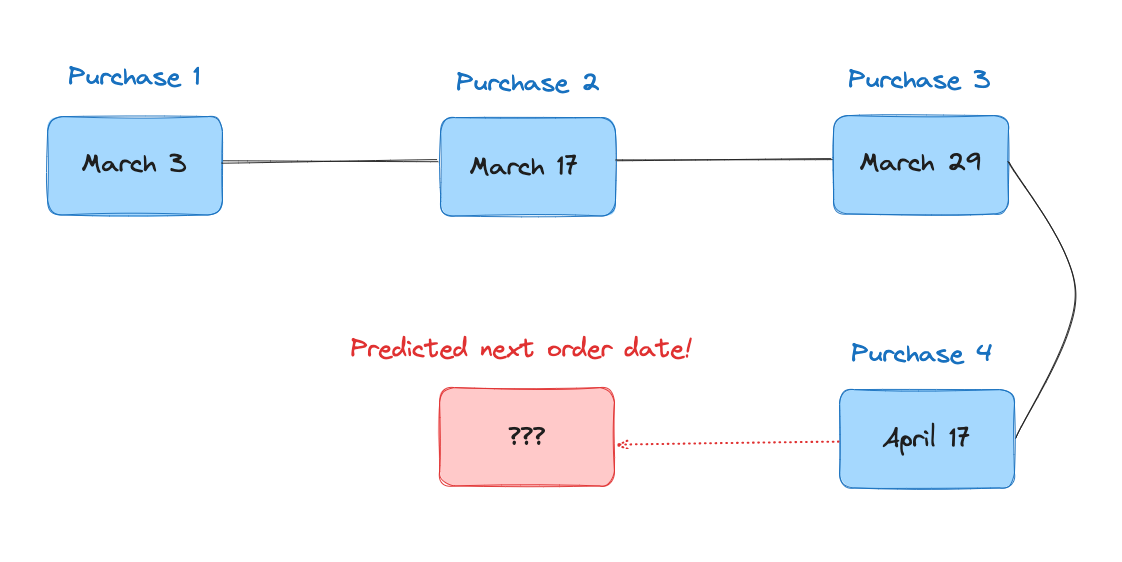
The time elapsed between each consecutive pair of purchases is 14 days, 12 days, and 19 days, respectively. To calculate the average, we simply add them and divide: 14+12+173=15 days. Using this result, we can make the simple guess that purchase 5 will be made fifteen days after April 17 on May 2.
This method can of course be generalized to more data points, more customers, and more complicated methods of statistical analysis beyond just taking an average, but the principle is still the same: by looking at a consumer’s order history and figuring out the average time it takes for them to make another purchase, you can make an educated guess for when they’ll buy something next.
What if someone has only made one purchase? Then you can use the data you’ve collected from all your other customers to create a general average. If the math works out so that the rest of your consumer base averages around two weeks between each purchase, you can be fairly confident that this will hold for any one consumer.
Storing and Activating This Information
You may be wondering where we can get all of this data on customers. Well, the methods for actually collecting that data can be another discussion entirely, but what helps make your data easy to manage and work with is how you store it. A common kind of data management system for the kind of predictive analytics we’re interested in is a data warehouse. In general, they’re characterized by the ability to store large amounts of historical data and their ability to perform queries—a request for a specific piece of information within that database.
Once you’ve consolidated all the data you received from multiple sources, it needs to be prepared for analysis in the data warehouse, which typically has an extraction system for this purpose: cleaning, transforming, and standardizing the data to ensure it’s consistent, usable, and useful. In our example above, the step where we calculated the time elapsed can occur during extraction, perhaps by creating a variable for the interval between two purchases. It may also help to pick through the data and identify what kinds of information are most helpful to predict the next customer purchase day: we probably care a little more about a customer’s responsiveness to marketing campaigns than we care about, say, the model of iPhone they’re using.
To make use of this data, the warehouse ideally has some statistical capabilities, like the step we took to calculate the average of the three intervals. On a more complex level, this can look like using regression analysis, customer segmentation, and even machine learning to produce a final estimation. After performing this analysis, it’s easier to get the “full picture” of your business so you can derive more valuable insights.
Bringing It All Together
So, you have a general idea of consumer behavior and the data and numbers to back it up. What now?
One very common step is integrating it into your business’s emailing habits. As we’ve mentioned several times throughout this article, emails are a valuable promotional tactic, and timing can be crucial when it comes to maximizing their efficacy: you don’t want to send an email that’ll just end up in the Trash folder. So we want to figure out how to transfer data from your warehouse to an email service provider (ESP) to make the most of your communication.
An ESP is a software platform that can be used to build customizable templates, send campaigns, and gather more data. Specifically, it can help segment your mailing list into groups of customers that share specific traits, so you can better tailor the emails you send. There are a variety of ways to transfer data into an ESP:
- A manual export/import works best for smaller data sets: simply download your data into something like a CSV file and upload it to your ESP.
- Some ESPs offer APIs that allow for automated data transfer. You can use external platforms or write your own scripts to complete the integration step.
- Other ESPs allow for real-time syncing through direct connections to your data warehouse. This is most effective for ongoing, constantly-updating campaigns.
Once your data is in the ESP, you’re ready to create and send your marketing campaigns out to the world! Most ESPs also offer metrics on the emails you send, such as open rates or click-through rates, which allow you to monitor the performance of specific campaigns or the responsiveness of certain customer groups. In general, although more automation and more complex transfer processes require more work to set up, they also enable you to quickly respond to these metrics and optimize your marketing, saving you resources in the long run.
Conclusion
Although email campaigns are one of the most common uses for predicting the next customer purchase day, there is similar value in applying this prediction to advertising campaigns, loyalty reward systems, and even pricing adjustments. Even simple calculations like taking an average can produce a result that has a significant impact on the way you structure your marketing strategies. Somewhere out there, I hope my Martinelli’s-obsessed friend is making a marketing analyst very happy.